[This post was written by PTJ]
One of the slightly disconcerting experiences from my week in Vienna teaching an intensive philosophy of science course for the European Consortium on Political Research involved coming out of the bubble of dialogues with Wittgenstein, Popper, Searle, Weber, etc. into the unfortunate everyday actuality of contemporary social-scientific practices of inquiry. In the philosophical literature, an appreciably and admirably broad diversity reigns, despite the best efforts of partisans to tie up all of the pieces of the philosophy of science into a single and univocal whole or to set perennial debates unambiguously to rest: while everyone agrees that science in some sense “works,” there is no consensus about how and why, or even whether it works well enough or could stand to be categorically improved. Contrast the reigning unexamined and usually unacknowledged consensus of large swaths of the contemporary social sciences that scientific inquiry is neopositivist inquiry, in which the endless drive to falsify hypothetical conjectures containing nomothetic generalizations is operationalized in the effort to disclose ever-finer degrees of cross-case covariation among ever-more-narrowly-defined variables, through the use of ever-more sophisticated statistical techniques. I will admit to feeling more than a little like Han Solo when the Millennium Falcon entered the Alderaan system: “we’ve come out of hyperspace into a meteor storm.”
Two examples leap to mind, characteristic of what I will somewhat ambitiously call the commonsensical notion of inquiry in the contemporary social sciences. One is the recent exchange in the comments section of PM’s post on Big Data (I feel like we ought to treat that as a proper noun, and after a week in a German-speaking country capitalizing proper nouns just feels right to me) about the notion of “statistical inference,” in which PM and I highlight the importance of theory and methodology to causal explanation, and Eric Voeten (unless I grossly misunderstand him) suggests that inference is a technical problem that can be resolved by statistical techniques alone. The second is the methodological afterword to the AAC&U report “Five High-Impact Practices” (the kind of thing that those of us who wear academic administrator hats in addition to our other hats tend to read when thinking about issues of curriculum design), which echoes some of the observations made in the main report on the methodological limitations of research on practices higher education such as first-year seminars and undergraduate research opportunities — what is called for throughout is a greater effort to deal with the “selection bias” caused by the fact that students who select these programs as undergraduates might be those students already inclined to perform well on the outcome measures that are used to evaluate the programs (students interested in research choose undergraduate research opportunities, for example), and therefore it is difficult if not impossible to ascertain the independent impact of the programs themselves. (There are also some recommendations about defining program components more precisely so that impacts can be further and more precisely delineated, especially in situations where a college or university’s curriculum contains multiple “high-impact practices,” but those just strengthen the basic orientation of the criticisms.)
The common thread here is the neopositivist idea that “to explain” is synonymous with “to identify robust covariations between,” so that “X explains Y” means, in operational terms, “X covaries significantly with Y.” X’s separability from Y, and from any other independent variables, is presumed as part of this package, so efforts have to be taken to establish the independence of X. The gold standard for so doing is the experimental situation, in which we can precisely control for things such that two populations only vary from one another in their value of X; then a simple measurement of Y will show us whether X “explains” Y in this neopositivist sense. Nothing more is required: no complex assessments of measurement error, no likelihood estimates, nothing but observation and some pretty basic math. When we have multiple experiments to consider, conclusions get stronger, because we can see — literally, see — how robust our conclusions are, and here again a little very basic math suffices to give us a measure of confidence in our conclusions.
But note that these conclusions are conclusions about repeated experiments. Running a bunch of trials under experimental conditions allows me to say something about the probability of observing similar relationships the next time I run the experiment, and it does so as long as we adopt something like Karl Popper’s resolution of Hume’s problem of induction: no amount of repeated observation can ever suffice to give us complete confidence in the general law (or: nomothetic relationship, since for Popper as for the original logical positivists in the Vienna Circle a general law is nothing but a robust set of empirical observations of covariation) we think we’ve observed in action, but repeated failures to falsify our conjecture is a sufficient basis for provisionally accepting the law. The problem here is that we’ve only gotten as far as the laboratory door, so we know what is likely to happen in the next trial, but what confidence do we have about what will happen outside of the lab? The neopositivist answer is to presume that the lab is a systematic window into the wider world, that statistical relationships revealed through experiment tell us something about one and the same world — a world the mind-independent character of which underpins all of our systematic observations — that is both inside and outside of the laboratory. But this is itself a hypothetical conjecture, for a consistent neopositivism, so it too has to be tested; the fact that lab results seem to work pretty well constitute, for a neopositivist, sufficient failures to falsify that it’s okay to provisionally accept lab results as saying something about the wider world too.
Now, there’s another answer to the question of why lab results work, which has less to do with conjecture and more to do with the specific character of the experimental situation itself. In a laboratory one can artificially control the situation so that specific factors are isolated and their independent effects ascertained; this, after all, is what lab experiments are all about. (I am setting aside lab work involving detection, because that’s a whole different kettle of fish, philosophically speaking: detection is not, strictly speaking, an experiment, in the sense I am using the term here. But I digress.) As scientific realists at least back to Rom Harré have pointed out, this means that the only way to get those results out of the lab is to make two moves: first, to recognize that what lab experiments do is to disclose cause powers, defined as tendencies to produce effects under certain circumstances, and second, to “transfactually” presume that those causal powers will operate in the absence of the artificially-designed laboratory circumstances that produce more or less strict covariations between inputs and outputs. In other words, a claim that this magnetic object attracts this metallic object is not a claim about the covariation of “these objects being in close proximity to one another” and “these objects coming together”; the causal power of a magnet to attract metallic objects might or might not be realized under various circumstances (e.g. in the presence of a strong electric field, or the presence of another, stronger magnet). It is instead not a merely behavioral claim, but a claim about dispositional properties — causal powers, or what we often call in the social sciences “causal mechanisms” — that probably won’t manifest in the open system of the actual world in the form of statistically significant covariations of factors. Indeed, realists argue, thinking about what laboratory experiments do in this way actually gives us greater confidence in the outcome of the next lab trial, too, since a causal mechanism is a better place to lodge an account of causality than a mere covariation, no matter how robust, could ever be.
Hence there are at least two ways of getting results out of the lab and into the wider world: the neopositivist testing of the proposition that lab experiments tell us something about the wider world, and the realist transfactual presumption that causal powers artificially isolated in the lab will continue to manifest in the wider world even though that manifestation will be greatly affected by the sheer complexity of life outside the lab. Both rely on a reasonably sharp laboratory/world distinction, and both suggest that valid knowledge depends, to some extent, on that separation. This impetus underpins the actual lab work in the social sciences, whether psychological or or cognitive or, arguably, computer-simulated; it also informs the steady search of social scientists for the “natural experiment,” a situation close enough to a laboratory experiment that we can almost imagine that we ran it in a lab. (Whether there are such “natural experiments,” really, is a different matter.)
Okay, so what about, you know, most of the empirical work done in the social sciences, which doesn’t have a laboratory component but still claims to be making valid claims about the causal role of independent factors? Enter “inferential statistics,” or the idea that one can collect open-system, actual-world data and then massage it to appropriately approximate a laboratory set-up, and draw conclusions from that.
Much of the apparatus of modern “statistical methods” comes in only when we don’t have a lab handy, and is designed to allow us to keep roughly the same methodology as that of the laboratory experiment despite the fact that we don’t, in fact, run experiments in controlled environments that allow us to artificially separate out different causal factors and estimate their impacts. Instead, we use a whole lot of fairly sophisticated mathematics to, put bluntly, imagine that our data was the result of an experimental trial, and then figure out how confident we can be in the results it generated. All of the technical apparatus of confidence intervals, different sorts of estimates, etc. is precisely what we would not need if we had laboratories, and it is all designed to address this perceived lack in our scientific approach. Of course, the tools and techniques have become so naturalized, especially in Political Science, that we rarely if ever actually reflect on why we are engaged in this whole calculation endeavor; the answer goes back to the laboratory, and its absence from our everyday research practices.
But if we put the pieces together, we encounter a bit of a profound problem: we don’t have any way of knowing whether these approximated labs that we build via statistical techniques actually tell us anything about the world. This is because, unlike an actual lab, the statistical lab-like construction (or “quasi-lab”) that we have built for ourselves has no clear outside — and this is not simply a matter of trying to validate results using other data. Any actual data that one collects still has to be processed and evaluated in the same way as the original data, which — since that original process was, so to speak, “lab-ifying” the data — amounts, philosophically speaking, to running another experimental trial in the same laboratory. There’s no outside world to relate to, no non-lab place in which the magnet might have a chance to attract the piece of metal under open-system, actual-world conditions. Instead, in order to see whether the effects we found in our quasi-lab obtain elsewhere, we have to convert that elsewhere into another quasi-lab. Which, to my mind, raises the very real possibility that the entire edifice of inferential statistical results is a grand illusion, a mass of symbols and calculations signifying nothing. And we’d never know. It’s not like we have the equivalent of airplanes flying and computers working to point to — those might serve as evidence that somehow the quasi-lab was working properly and helping us validate what needs to be validated, and vice versa. What we have is, to be blunt, a lot of quasi-lab results masquerading as valid knowledge.
One solution here is to do actual lab experiments, the results of which could be applied to the non-lab world in a pretty straightforward way whether one were a neopositivist or a realist: in neither case would one be looking for covariations, but instead one would be looking to see how and to what degree lab results manifested outside of the lab. Another solution would be to confine our expectations to the next laboratory trial, which would mean that causal claims would have to be confined to very similar situations. (An example, since I am writing this in Charles De Gaulle airport, a place where my luggage has a statistically significant probability of remaining once I fly away: based on my experience and the experience of others, I have determined that CDG has some causal mechanisms and process that very often produce a situation where luggage does not make it on to a connecting flight, and this is airline-invariant as far as I can tell. It is reasonable for me to expect that my luggage will not make it into my flight home, because this instance of my flying through CDG is another trial of the same experiment, and because so far as I know and have heard nothing has changed at CDG that would make it any more likely that my bag will make the flight I am about to board. What underpins my expectation here is the continuity of the causal factors, processes, and mechanisms that make up CDG, and generally incline me to fly through Schipol or Frankfurt instead whenever possible … sadly, not today. This kind of reasoning also works in delimited social systems like, say, Major League Baseball or some other sport with sufficiently large numbers of games per season.) Not sure how well this would work in the social sciences, unless we were happy only being able to say things about delimited situations; this might suffice for opinion pollsters, who are already in the habit of treating polls as simulated elections, and perhaps one could do this for legislative processes so long as the basic constitutional rules both written and unwritten remained the same, but I am not sure what other research topics would fit comfortably under this approach.
[A third solution would be to say that all causal claims were in important ways ideal-typical, but explicating that would take us very far afield so I am going to bracket that discussion for the moment — except to say that such a methodological approach would, if anything, make us even more skeptical about the actual-world validity of any observed covariation, and thus exacerbate the problem I am identifying here.]
But we don’t have much work that proceeds in any of these ways. Instead, we get endless variations on something like the following: collect data; run statistical procedures on data; find covariation; make completely unjustified assumption that the covariation is more than something produced artificially in the quasi-lab; claim to know something about the world. So in the AAC&U report I referenced earlier, the report’s authors and those who wrote the Afterword are not content with simply content to collect examples of innovative curriculum and pedagogy in contemporary higher education; they want to know, e.g., if first-year seminars and undergraduate research opportunities “work,” which means whether they significantly covary with desired outcomes. So to try to determine this, they gather data on actual programs … see the problem?
The whole procedure is misleading, almost as if it made sense to run a “field experiment” that would conduct trials on the actual subjects of the research to see what kinds of causal effects manifested themselves, and then somehow imagine that this told us something about the world outside of the experimental set-up. X significantly covarying with Y in a lab might tell me something, but X covarying with Y in the open system of the actual world doesn’t tell me anything — except, perhaps, that there might be something here to explain. Observed covariation is not an explanation, regardless of how complex the math is. So the philosophically correct answer to “we don’t know how successful these programs are” is not “gather more data and run more quasi-experiments to see what kind of causal effects we can artificially induce”; instead, the answer should be something like “conceptually isolate the causal factors and then look out into the actual world to see how they combine and concatenate to produce outcomes.” What we need here is theory and methodology, not more statistical wizardry.
Of course, for reasons having more to do with the sociology of higher education than with anything philosophically or methodologically defensible, academic administrators have to have statistically significant findings in order to get the permission and the funding to do things that any of us in this business who think about it for longer than a minute will agree are obviously good ideas, like first-year seminars and undergraduate research opportunities. (Think about it. Think … there, from your experience as an educator, and your experience in higher education, you agree. Duh. No statistics necessary.) So reports like the AAC&U report are great political tools for doing what needs to be done.
And who knows, they might even convince people who don’t think much about the methodology of the thing — and in my experience many permission-givers and veto-players in higher education don’t think much about the methodology of such studies. So I will keep using it, and other such studies, whenever I can, in the right context. Hmm. I wonder if that’s what goes on when members of our tribe generate a statistical finding from actual-world data and take it to the State Department or the Defense Department? Maybe all of this philosophy-of-science methodological criticism is beside the point, because most of what we do isn’t actually science of any sort, or even all that concerned with trying to be a science: it’s just politics. With math. And a significant degree of self-delusion about the epistemic foundations of the enterprise.
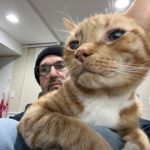
Daniel H. Nexon is a Professor at Georgetown University, with a joint appointment in the Department of Government and the School of Foreign Service. His academic work focuses on international-relations theory, power politics, empires and hegemony, and international order. He has also written on the relationship between popular culture and world politics.
He has held fellowships at Stanford University's Center for International Security and Cooperation and at the Ohio State University's Mershon Center for International Studies. During 2009-2010 he worked in the U.S. Department of Defense as a Council on Foreign Relations International Affairs Fellow. He was the lead editor of International Studies Quarterly from 2014-2018.
He is the author of The Struggle for Power in Early Modern Europe: Religious Conflict, Dynastic Empires, and International Change (Princeton University Press, 2009), which won the International Security Studies Section (ISSS) Best Book Award for 2010, and co-author of Exit from Hegemony: The Unraveling of the American Global Order (Oxford University Press, 2020). His articles have appeared in a lot of places. He is the founder of the The Duck of Minerva, and also blogs at Lawyers, Guns and Money.
Admin do you want unlimited content for your wp blog?
Serarch in google:
Anightund’s rewriter